Research
What makes individuals vulnerable to depression, anxiety, and related disorders?
​
Depression and anxiety are leading causes of disability globally. Their frequent co-occurrence suggests they may share common causes and risk factors, but these are not fully understood. Some individuals with these debilitating internalizing disorders also experience other serious mental illnesses, such as substance use disorders and bipolar disorders. However, what contributes to these different patterns of co-occurrence still remains unclear.
Understanding crucial processes underlying depression, anxiety, and other related disorders, and identifying when and how these processes lead to different patterns of comorbidity, will be essential for refining current interventions and developing more effective treatment strategies.​
​​
Our Research Goals
​
The primary goal of our research is to identify cognitive-affective processes that confer risk for the development and maintenance of depression, anxiety, and co-occurring problems (e.g., harmful substance use, mania). We also aim to shed light on whether and how contextual factors (e.g., stress, trauma, social/family environments) shape, alter, and/or interact with these cognitive-affective processes. By doing so, we seek to better characterize individuals who are most vulnerable and would benefit most from targeted intervention and prevention efforts.​
Questions we aim to answer include:
-
How and when does emotion regulation (i.e., the way we monitor and modify our own emotions) contribute to depression, anxiety, and co-occurring problems?
-
How do contextual factors influence the relationship between emotion regulation and these disorders?
-
What factors contribute to or hinder the development and refinement of emotion regulation abilities and strategies?
-
How and when do aberrations in the way we respond to reward and threat (i.e., reward/threat sensitivity) confer risk for depression, anxiety, and co-occurring problems?
-
How are deficits in positive and negative emotion regulation commonly and uniquely associated with these disorders?
-
Do aberrations in reward and threat sensitivity contribute to depression, anxiety, and co-occurring problems through deficits in emotion regulation?
Methods
We use multiple research and advanced statistical methods to better capture the complex and dynamic associations between cognitive-affective processes, contextual factors, and various forms of psychopathology both within and across individuals. The research and statistical methods we use include:


Electroencephalography (EEG) & Event-Related Potentials (ERPs)
​
EEG measures the brain’s electrical activity using sensors placed on the scalp. ERPs are extracted from the continuous EEG data and represent voltage changes that are synchronized with specific events, such as the onset of a stimulus. Since electrical signals travel almost at the speed of light, the activity detected by the scalp sensors occurs nearly simultaneously with the brain’s neural response. This makes ERPs an excellent tool for studying cognitive-affective processes with millisecond accuracy and monitoring changes in these processes in real-time (Weinberg & Park, 2024).
​
Experience Sampling Method (ESM)
​
ESM, or intensive longitudinal methods, involve repeated assessments of each individual participating in a given study. Compared to cross-sectional retrospective measures, ESM offers better ecological validity, as it more accurately reflects an individual’s real-life experiences and the variation in those experiences over time. ESM also provides more precise measurements by reducing recall biases that are common in responses to retrospective questionnaires. Repeated assessments in ESM are particularly effective in capturing processes like emotion regulation, which is viewed as an ongoing, context-dependent process that unfolds over time within individuals (Park & Naragon-Gainey, 2019).

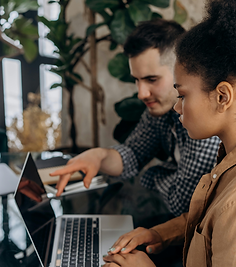
Multilevel Models
Multilevel models are a type of data analysis technique designed to handle ‘clustered’ or ‘nested’ data, which refers to data with a hierarchical structure. For example, in intensive longitudinal studies, measurement occasions are nested within individuals. It is crucial to account for the dependencies within clustered data, as failing to do so can lead to biased results, such as inflated Type I error and incorrect confidence intervals. Multilevel models also allow for the analysis of variables at different levels, offering greater flexibility and requiring fewer assumptions than traditional statistical methods (Geiser, 2013).
​​
​Mixture Models
Mixture models are a ‘person-centered’ approach used to examine similarities and differences among individuals in terms of how variables relate to one other within groups of individuals. This approach is based on the assumption that a population is heterogeneous, consisting of distinct subgroups that share common characteristics. Examples include latent class analysis and latent profile analysis, which identify latent groups in data that share meaningful and interpretable patterns of responses on the measures of interest. Mixture models are particularly useful for uncovering patterns of shared behavior or psychological processes both between and within samples (See Park & Naragon-Gainey, 2024; Park, Banica, & Weinberg, 2023 for examples).